HOW AI-POWERED DRUG DEVELOPMENT IS RESHAPING PHARMACEUTICAL RESEARCH
This is a post from the Visium Blog by Matteo Togninalli, Chief Operating Officer
Aug 28, 2023 • 12 min. read
Generative AI hasGenerative AI hastaken the world by storm. Significant advances have been made in the field of AI over the past two decades, and it is now routinely applied in many industries. Current AI applications cover a broad range of activities, including image recognition, mining of large and unstructured datasets, personalized learning, and many others. Breakthroughs in large language models, such as GPT-4, have certainly redefined human-computer interactions.Drug development isDrug development isno exception to these broader trends seen in AI, especially given the steep rise of development costs for new drugs.
For every new drug brought to the market, it costs $6.16 Billion and takes more than 10 years of work. Hence, pharma companies are always interested in new strategies that can facilitate and accelerate the drug development process.
In this article, we cover the AI uses within the drug development process, detail the key challenges and opportunities within the biopharmaceutical industry, and provide real-world examples of AI in action.
UNDERSTANDING DRUG DEVELOPMENT
The process of drug development involves introducing a novel drug molecule into clinical usage. In its broadest sense, this encompasses all stages, starting from the initial research to identify a suitable molecular target, right up to supporting the drug's launch into the market.
Creating and introducing a new drug to the market is time-consuming and costly. The success of a new drug hinges greatly upon robust cooperation and collaboration among many departments within the drug development organization, external investigators and service providers, in constant dialogue with regulatory authorities, academic experts, clinicians, and patient organizations. Across the different phases of a drug's lifecycle, drug development stands out as the most pivotal aspect for both its initial and sustained triumph in the market.
The estimated expense for research and development associated with each effective drug has reached $6.16 Billion in 2023. This financial estimate encompasses the costs incurred due to the numerous failures encountered along the way. From every 5,000 to 10,000 compounds that enter the evaluation and development pipeline, only one ultimately secures approval.
These figures might seem astounding, but a brief understanding of the research and development process can shed light on why a multitude of compounds fail to progress and why a substantial effort is required to bring a single medicine to patients.
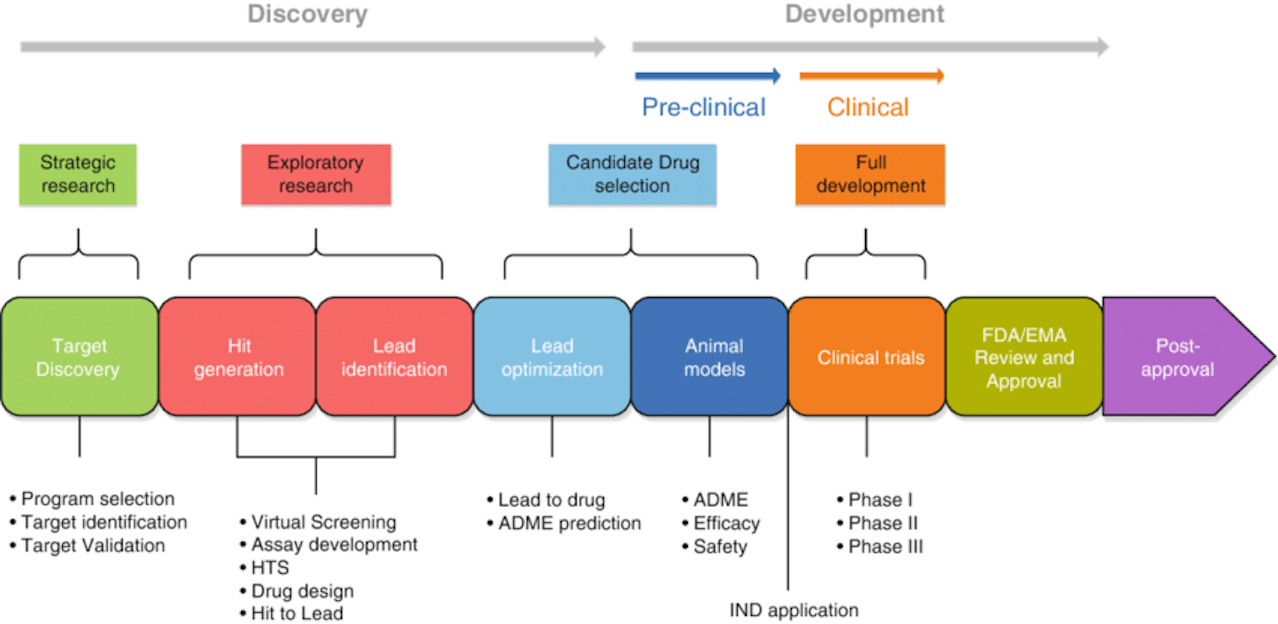
ADVANCING DRUG DEVELOPMENT WITH AI
The high costs, delays, and failures in clinical trials all negatively affect patients. Despite many advancements, a large number of diseases still lack effective drug treatments. This makes finding better and faster ways to conduct clinical trials extremely important.
A notable point is that AI is more commonly used in discovering new drugs compared to its use in clinical development. This is due to data sets being more easily available in drug discovery, but also because drug discovery involves testing potential new drugs on cells and animals, and doesn't have the same strict regulations as testing on people. Yet, more and more academic institutions, biopharma companies, and smaller biotech firms are realizing the potential of AI to revolutionize clinical trials.
AI can transform various important stages of clinical trials, from planning to execution, leading to better success rates and reducing the challenges of biopharma research and development.
In the following sections, we explore different AI use cases in the drug development process.
1. STUDY DESIGN
Biopharmaceutical companies are adopting various approaches to innovate clinical trials. To achieve effectiveness, these strategies rely on increasing volumes of scientific and research data from diverse sources like past and ongoing clinical trials, patient support programs, and post-market surveillance. AI applied to real-world data (RWD) can extract meaningful patterns from this information, enhancing the design of clinical trials.
Moreover, AI technology and natural language processing can be utilized to determine and select the optimal primary and secondary endpoints in research design to ensure that the most pertinent protocols are established using healthcare data sets. A more precise study design enables shorter protocol development cycles, more predictable findings, improved recruitment rates, and greater efficiency throughout the trial.
As an example, we can look at our partnership with a leading pharma research company using advanced RWE analytics to uncover links between insomnia, treatments, and daytime impairments. We built a data-driven ontology to characterize a specific disease area and applied it to better understand real-world patient outcomes. Through advanced RWE analytics, the company acquired data from 80,000 patients within a mere 4.5 hours, sidestepping time-consuming chart reviews. This resulted in increased data quality and reliability of the resulting studies, matching the reliability of clinical trials.
2. CONDUCT OF CLINICAL TRIALS
In the last few years, quite a few innovative solutions have emerged for improving the conduct of clinical trials.
One of those is Synthetic Control Arms. SCAs are external control arms in which researchers use data to construct a virtual or synthetic control rather than recruiting new patients for a control group. Building an SAC involves utilizing patient data found in pre-existing datasets, such as electronic health records (EHR), patient-generated data from fitness trackers, or home medical equipment, that is stripped of any personally identifiable information. This data is then used to model or simulate the expected results, comparing them with those on the clinical trial. Eliminating or reducing the need for normal control arms ensures that all participants receive the active treatment, eliminating concerns about treatment assignment. Moreover, synthetic control arms can increase efficiency, reduce delays, lower trial costs and accelerate market entry for therapies.
Another recent addition to the innovation landscape are digital endpoints. When seeking FDA approval for a new drug, manufacturers are required to furnish substantial evidence of its clinically meaningful impact on patients. This evidence is often based on endpoints such as survival or significant biomarker reductions like LDL cholesterol or hemoglobin A1c.
Digital endpoints represent the latest evolution in this domain. These endpoints are evaluated using data collected by sensors, typically outside of clinical settings and during daily routines. These sensors usually can take the form of wearables, such as accelerometers in smartwatches capturing motion data.
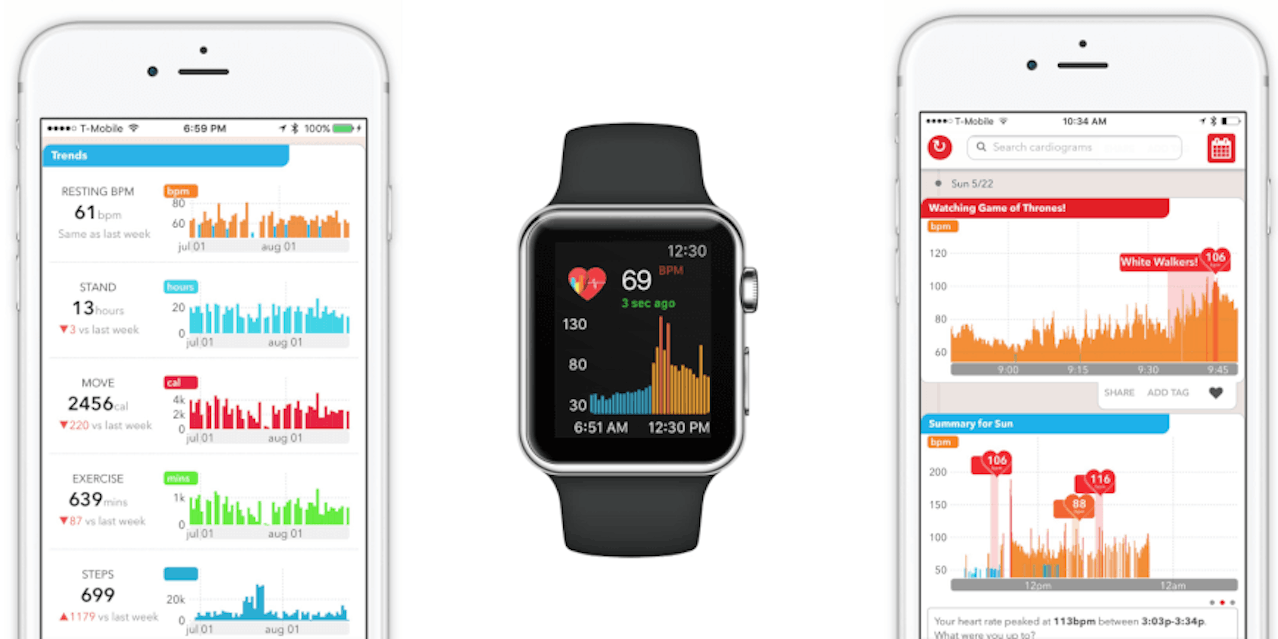
Cardiogram
Wearable devices employ disease-specific algorithms to process participant data, yielding more precise insights into digital biomarkers. In a Parkinson's disease study, tailored algorithms might quantify symptom severity and progression, including involuntary movements, tremors, and gait patterns.
While conventional endpoints and biomarkers are still necessary for FDA submissions and regulations, digital biomarkers offer sponsors contextual information, empowering confident and efficient decision-making. For instance, innovative digital biomarkers could signal potential phase III trial failure, which may go undetected by traditional endpoints.
3. PATIENT RECRUITMENT
Finding suitable trial sites to effectively conduct clinical research with access to enough eligible patients poses an ongoing challenge. As studies target narrower patient populations, achieving recruitment goals becomes even more difficult, leading to increased costs, longer timelines, and higher chances of failure.
Research shows thatResearch shows that37% of sites do not meet their enrollment target, while 11% of sites fail to enroll a single patient. AI and machine learning (ML) can help mitigate these challenges by identifying sites with the best recruitment potential and suggesting appropriate recruitment strategies. This involves mapping patient populations and proactively targeting sites with high predicted potential for recruiting the most patients – even before any site is opened – and identifying optimal approaches to attract them.
One of the most advanced parts of utilizing AI in patient identification and recruitment is through analyzing social media content. AI can be used to mine through online forums where patients exchange information about their conditions to detect if there are certain locations or regions where a medical condition might be more prevalent. This approach helps clinical trial organizers to speed up cohort identification, which would help companies design clinical trials effectively.
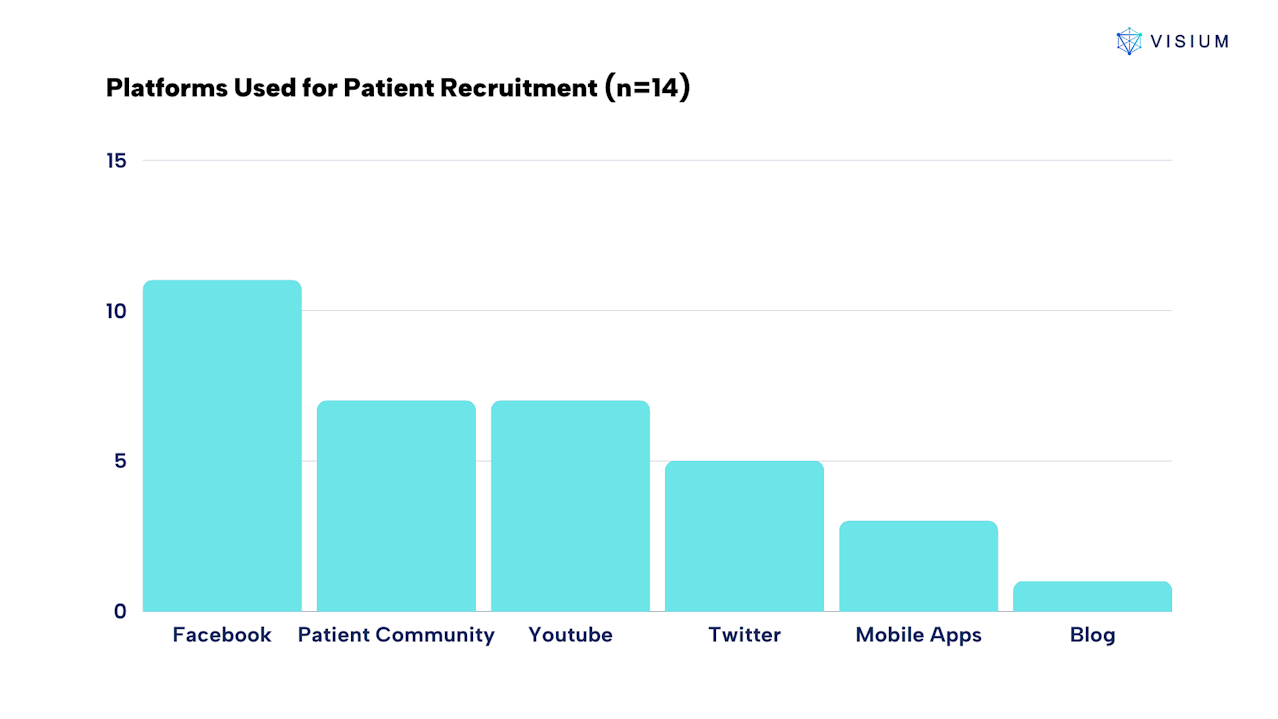
Tufts CSDD
This approach has already been tested in a neurological study aiming to identify patients with Alzheimer’s disease after receiving interest from potential study populations that did not have a clear diagnosis. By using predictive technology and analysis of digital biomarkers in the cohort’s behaviour on social media, individuals diagnosed with Alzheimer's disease were accurately identified, along with predictions of their geographic locations.
4. CLINICAL MONITORING
Algorithms can also play a role in monitoring and overseeing patients by automating data capture, digitizing standard clinical assessments, and sharing data across various systems. Moreover, AI solutions can aid nurses and physicians in determining necessary actions as per protocol requirements, including specific clinical tests and procedures for monitoring diagnostic biomarkers, aiding in scheduling patient visits, and pre-populating patient data into EDC systems.
During most clinical trials, researchers' insight into patients' health is limited to scheduled site visits. Accurately gauging patient adherence to the treatment plan is thus challenging. This disparity can lead to differences between treatment efficacy in clinical trials and real-world drug effects. Advanced AI algorithms, utilizing data collected through wearables, apps, and sensors from trial participants, can offer real-time insights into treatment safety and effectiveness. Importantly, these connected apps and devices enable patients to receive real-time information and support, potentially enhancing engagement and retention.
One of the initiatives that is on the way to disrupt the status quo is Novartis FocalView app, launched in 2018. The app was developed through Apple’s ResearchKit to be used as an ophthalmic digital research platform and it aims to make ophthalmology clinical trials more accessible and flexible by allowing clinical researchers to monitor disease progression by collecting self-reported data in real time directly from consenting patients. This app was launched in the App Store in the US to be tested in a prospective, non-interventional study to assess its ease of use and ability to collect important clinical data and other documentation, including informed consent.
CHALLENGES IN AI ADOPTION INBIOPHARMA
The integration of AI holds tremendous promise for revolutionizing the biopharma industry. However, alongside this enthusiasm, there are intricate challenges that demand careful consideration and strategic solutions.
THE NEED FOR RELIABLE DATA
AI technologies need reliable data to train their algorithms effectively and make users feel confident in how well the technology works. This data must be identified, prepared, and stored in a way that makes it easy to analyze. However, the large amounts of data produced by the biopharmaceutical industry are often messy, which makes it harder to use effectively. The quality and dependability of data sources can vary a lot, and the data might be in the form of text, audio, video, or images, which current AI technologies sometimes struggle to understand without help from skilled humans.
With data being the new currency in the field of life sciences, biopharma companies are in a race to get as much data as they can. They do this by partnering, collaborating, and merging with other companies, or building their own capabilities. It's important for biopharma companies to ensure that any patient data they use is allowed for the specific purpose and kept private and safe. It's also crucial to improve how information is shared between everyone involved about problems, incidents, risks, best practices, and strategies to deal with them.
The good news is that a novel AI training approach, known as federated learning, is poised to simplify the process of training models with extensive data from diverse geographical, racial, and socio-economic backgrounds. With federated learning, researchers send their AI model to external data sites—such as universities or healthcare systems—and simply collect back the weighted scores once the model has been trained on the external data. As a result, the data remains with its respective owners, streamlining compliance with data privacy regulations, minimizing administrative steps, and reducing data acquisition expenses.
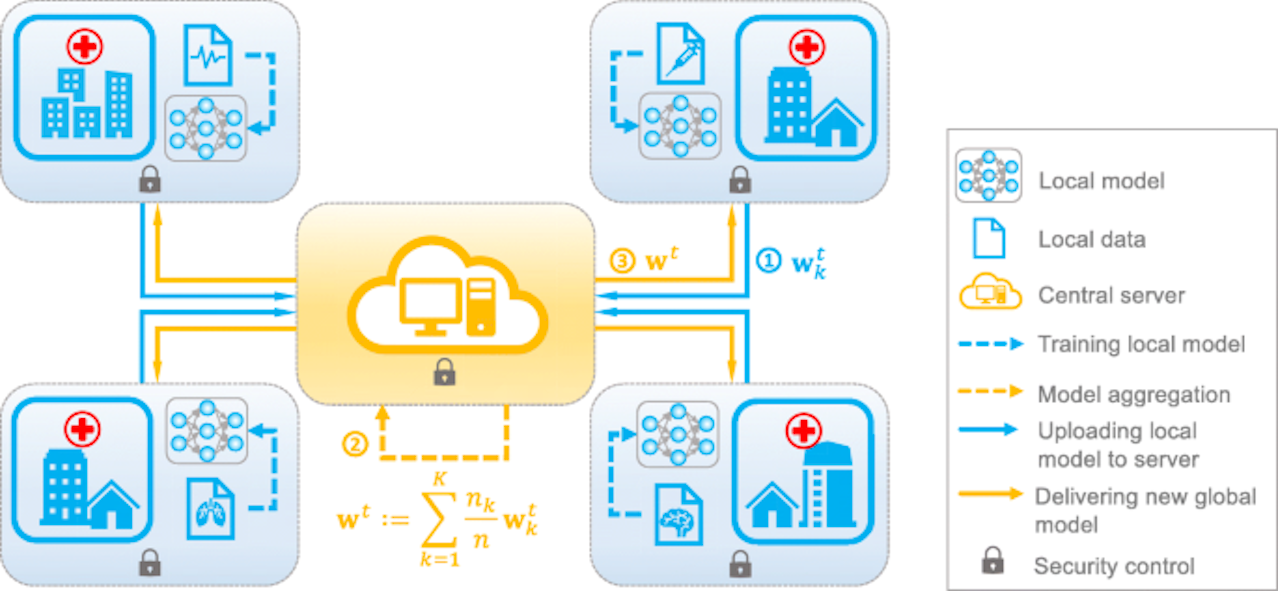
Xu, J., Glicksberg, B.S., Su, C. et al. Federated Learning for Healthcare Informatics.
It's important to recognize that federated learning is not without its limitations. In optimal scenarios where data is independently and identically distributed (iid), federated learning performs on par with ensemble models. However, data segmented along national boundaries lacks the iid property due to significant differences in patients and healthcare systems across countries. Each dataset retains biases within its silo and exports these biases to all ensembles via shared models.
For example, in 2019 the age-standardized share of adults with diabetes was 3.2% in Ireland, and 10.4% in Germany. An FL model ensemble covering both countries may over-diagnose diabetes in Ireland and under-diagnose diabetes in Germany. Numerous solutions have been suggested to address the non-iid data distribution challenge, yielding differing degrees of effectiveness; nonetheless, these enhancements have yet to achieve the precision achieved by a centralized ML framework.
UPGRADING DATA AND IT INFRASTRUCTURE
AI technologies rely on data for their learning process, and the advancements in computer systems and the underlying infrastructure responsible for executing algorithms — encompassing hardware, software, and services — play a pivotal role in facilitating the growth of AI. The implementation of AI technologies necessitates setup, training, and assistance.
Introducing and integrating AI hardware and software into a biopharmaceutical company's existing IT framework can be a complex task that demands specialized expertise, a resource currently deficient in numerous biopharma companies. Similarly, the provision of support and maintenance services to uphold or upgrade the IT infrastructure to an acceptable level can also pose challenges in terms of costs and the time required to keep up to date.
ETHICAL CONSIDERATIONS
While AI can make clinical trials faster, cheaper, and more accurate, it’s easy to overlook its ethical implications in the excitement over the potential. One pressing concern revolves around data privacy. As AI models require substantial amount of data to work reliably, training AI models usually entail providing them with large quantities of medical records that are consolidated into a unified database for analysis. Yet, housing such a vast repository of sensitive information entails substantial risks to cybersecurity and privacy.
The potential for bias in AI models presents another ethical issue. If the training data contains inherent biases, the AI algorithms can learn and perpetuate these biases in decision-making processes. In the context of drug development, biased training data might lead to skewed predictions or recommendations, affecting treatment outcomes and patient care.
Lastly, the "black box" nature of some AI systems can undermine transparency, making it difficult to explain how the model uses data. This hinders informed consent in clinical trials, highlighting the need for enhanced transparency measures to foster trust and accountability.
NAVIGATING REGULATIONS
The biopharma industry operates in an increasingly complex regulatory landscape. Over the past few years, there has been a notable rise in regulatory modifications within the industry, with numerous upcoming regulations yet to be enacted. At the same time, regulatory authorities encounter the task of protecting the patients and advancing public health while fostering innovation and resourcefully adapting to the rapid advancements in the fields of medicine, science, and technology.
CASE STUDY: HOW MACHINELEARNING CAN HELP REDUCEBOTTLENECKS IN CLINICAL TRIALS
So far, we've seen that the use of AI in drug development has a lot of potential and opportunities. But how exactly are businesses leveraging it? It's time to explore a real-world example that bring the theoretical into the practical realm.
Preclinical studies generate high volumes of pathological data, making pathological analysis time-consuming. To comply with rigorous drug safety standards, our client, a global pharma company, analyzes thousands of whole slide images as part of the toxicity assessment process. In each study, pathologists must find and score subtle lesions on approximately 1600 slides, each measuring 200 million pixels (i.e. 23 meters by 23 meters). However, approx 70% of the slides do not contain any lesions. So most of the time pathologists are looking at normal slides.
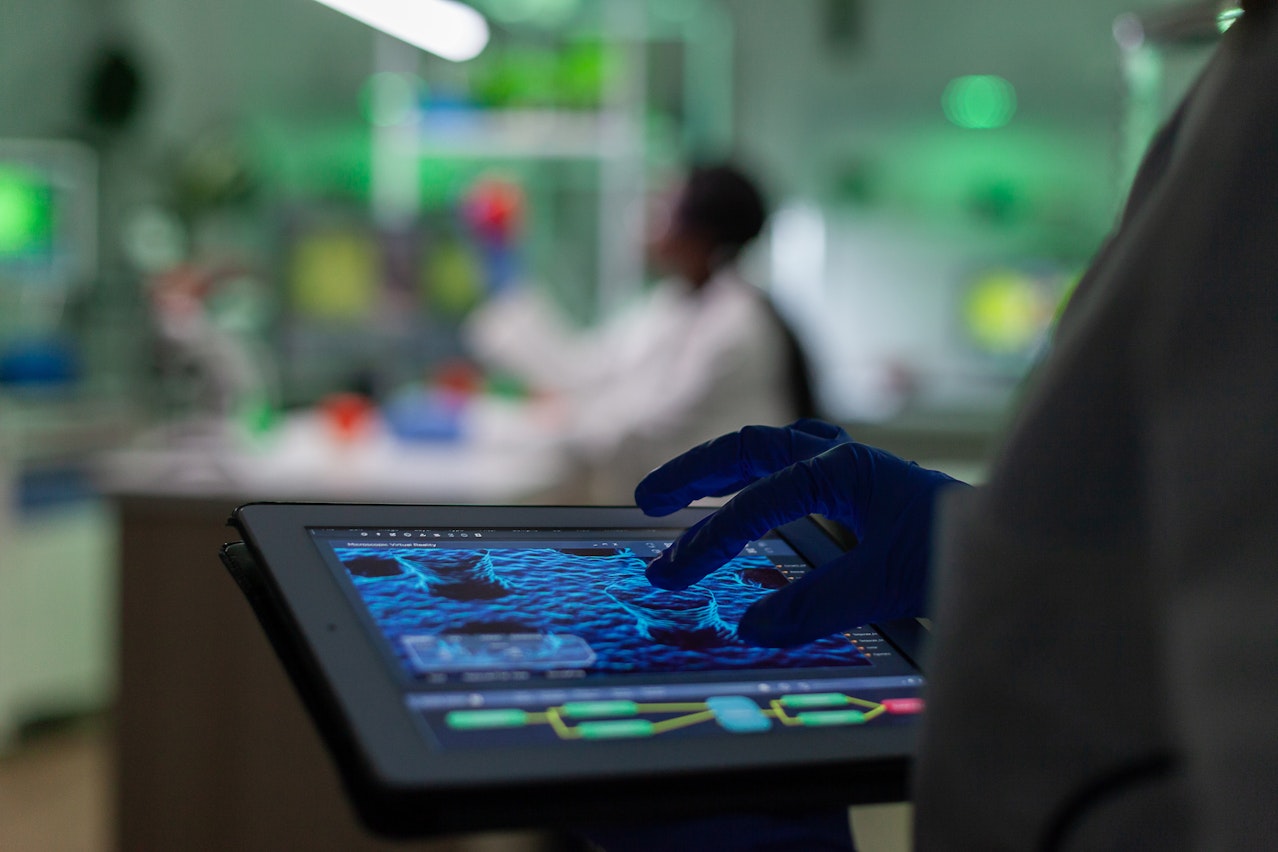
Together, we developed a Machine Learning model that identifies healthy slides and discards them automatically using the latest computer vision techniques and digital pathology data. The model provides heatmaps on tissue slides to highlight lesion-prone areas for expert pathologists, making their analysis more accurate and efficient. For a more comprehensive understanding, check out this video.
AI-POWERED FUTURE OF DRUG DEVELOPMENT
Artificial intelligence is already transforming the drug development value chain, offering immense potential to increase efficiency, reduce costs, and speed up the discovery and development of life-saving drugs.
And while some pharma companies untangle AI's benefits quicker than others, by finding the balance between recognizing AI technologies' opportunities and its potential risks, it's only a matter of time until it is fully integrated into the drug development process.