How AI-driven digital biomarkers are redefining diagnostics
How AI-driven digital biomarkers are redefining diagnostics admin
Quick Takes
- Digital biomarkers are based on an algorithm or pattern derived from data that correlates to a specific disease or condition
- Benefits of digital biomarkers include improving automated recognition of signs of disease, enhancing clinical trial enrollment and patient assessments, and increasing diagnostic reliability
- Adoption of digital biomarkers will depend on their ability to demonstrate safety, cost-effectiveness, and their ease of integration into current processes
Digital biomarkers are playing an increasingly important role in the way patients receive and experience care. The longitudinal and continual data points that can be collected through different sources, including wearables, are making it possible for physicians to provide more timely and personalized treatment plans for their patients. Backed by artificial intelligence (AI), digital biomarkers could radically alter the kinds of outcomes patients are able to achieve in the near future.
We spoke with Joshua Reicher and Michael Muelly, cofounders of IMVARIA, a US-based company specializing in the development of AI-driven digital biomarker solutions, to learn about the impact of digital biomarkers on diagnostics and prognostics, especially in the area of rare diseases, and how they are being used to improve patient health.
Digital biomarkers: Using algorithms for identifying patterns
HT: What are digital biomarkers? Can you provide some examples of how they are currently transforming the healthcare industry?
Joshua Reicher: A formal definition of a biomarker is a biological molecule found in bodily fluids, blood, or tissues that signifies normal or abnormal processes, or is associated with a condition or disease. To expand upon this concept, the digital biomarker refers to digital signatures which do not necessarily come from blood, fluids, or tissues but are a sign of a normal or abnormal process related to a condition or disease.
These digital biomarkers are derived from wearables and technologies such as computerized tomography (CT) scans or magnetic resonance imaging (MRI), but they cannot be determined by manual review of raw data alone. Machine learning and AI must be used to find an algorithm or pattern that has a connection to a certain condition.
Michael Muelly: There are an increasing number of digital biomarker products available in the healthcare industry. One example of this is Fractional Flow Reserve – Computed Tomography (FFRCT) from HeartFlow which quantifies heart disease and avoids unnecessary invasive treatments. Additionally, electrocardiogram patch technologies have started defining categories such as atrial fibrillation burden where a digital biomarker can be determined by correlating it to a familiar disease and predicting outcomes based on it.
HT: So, in summary, a digital biomarker is more than just data, it’s rather the patterns and algorithms that define it. Is it then possible to have the same biological signal monitored by two independent biomarkers that lead to two different pieces of information?
Joshua Reicher: Yes, absolutely. In general, we can think about it in terms of what clinicians do. As a radiologist analyzing a CT scan, I can come up with different information and insights from one scan. To be more specific, I can assess the severity of a patient’s chronic obstructive pulmonary disease (COPD) or the presence of cancer. In the same manner that we test blood and tissues, the actual signature will depend on what you’re expecting. The raw data alone is what feeds into all of these things.
For biomarkers that signature needs to be correlated to something concrete, like a diagnosis or treatment assignment outcome. It cannot just be a raw piece of information or data on its own.
Adding value to diagnostics with digital biomarkers
HT: Your focus is on developing digital biomarkers for diagnostics. What is the value of digital biomarkers in diagnostics, specifically for rare diseases?
Joshua Reicher: There are two broad categories for describing the value of digital biomarkers in diagnostics. The first category is earlier disease detection. This is particularly true in challenging diseases, with rare diseases being the greatest example. While individually rare diseases by definition impact relatively few individuals, in aggregate, these diseases affect more than 30 million patients in the US.1 In some cases, it can take over seven years for a patient to receive the correct diagnosis of a rare disease.2 That’s an incredibly long period of time when a patient is bouncing around health systems, trying to find a doctor that can understand what’s going on before they finally reach a diagnosis. Solving that problem can lead to better patient journeys, faster pathways to accurate diagnosis, and of course, in many cases, more effective treatments.
Michael Muelly: The second category is misdiagnosis, which is something many people have experienced. Misdiagnoses are resulting in about $100 billion in annual costs in the US alone.3 Overly invasive testing is part of this, and inappropriate testing is another. With rare disease diagnoses, the major challenge is the countless misses, mischaracterizations, incorrect phenotyping, and inability to figure out what to do next.
Many patients have had these experiences with the health system, where they haven’t gotten to the correct, reliable diagnosis efficiently. Digital biomarkers, which can be made widely available and can be automated, can solve many of these problems because they are more objective and consistent.
Improving patient outcomes with digital solutions
HT: How will digital biomarkers potentially lead to better patient outcomes?
Joshua Reicher: If we take a look at the history of medicine, before CT scans, blood tests, imaging, and blood biomarkers, diseases were diagnosed by highly trained medical experts and there were several challenges. For example, obtaining accurate patient information and histories was difficult and subjective due to the different experiences doctors had had. And often invasive procedures at specialized medical institutions were required. Even something as simple as diagnosing gallstones required surgery.
Today, however, digital biomarkers provide objective, widely available systems to assess a wide range of diseases. Early and accurate diagnoses mean patients have a much better chance of getting their condition under control and ultimately achieving much better outcomes.
Our team at IMVARIA is examining our digital biomarker technology in lung fibrosis. The average delay in diagnosis is two years.4 During this time a patient can lose 30-40% of their lung function.5
Michael Muelly: Digital biomarkers also allow us to create personalized medicines by capturing individual patient characteristics and by comparing them at a population level by considering statistical averages. Digital biomarkers help us make critical inferences, which will hopefully be the difference in patient outcomes.
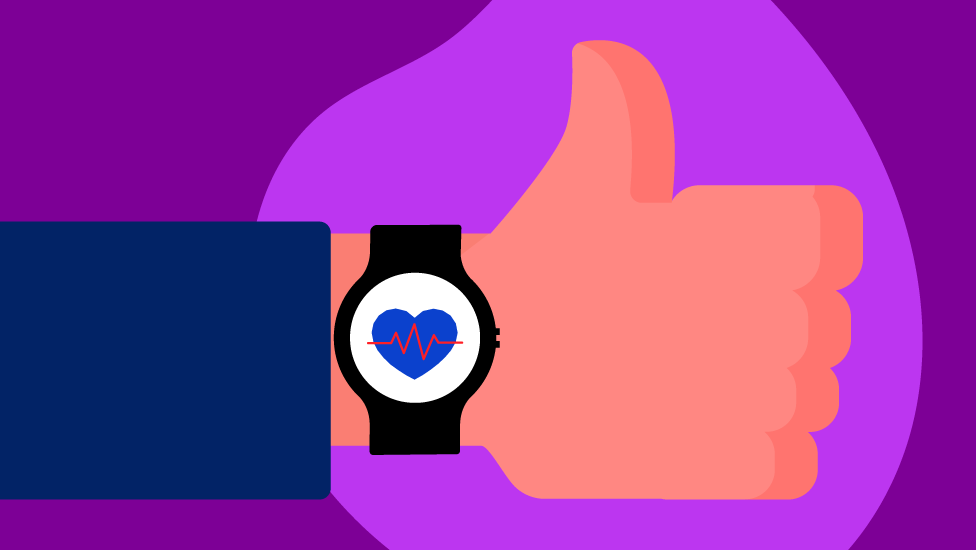
The benefits that digital biomarkers bring to healthcare systems
HT: What are some resource efficiency gains and/or financial benefits of digital biomarkers for healthcare systems? Can you give any specific examples?
Joshua Reicher: Yes, let’s take open-surgery biopsies, which are the current standard for diagnosing lung fibrosis. They come with high hospitalization costs that can be as much as $USD 30,000.6 A non-invasive digital biomarker that could answer the same questions, could reduce costs by an average of 55%, according to our data.7
Another example is HeartFlow.8 Previously released health economic studies show healthcare systems could achieve substantial savings from unnecessary invasive cardiac tests. 9
Ultimately, increasing the use and availability of digital biomarkers is something that needs to happen. Considering the cost and quality of today’s healthcare system, taking advantage of advanced AI technologies as well as developing digital biomarkers for more accurate yet less invasive diagnostics is an essential step forward.
Addressing the challenges with digital biomarker adoption
HT: What challenges are digital biomarker developers currently facing?
Michael Muelly: One of the main obstacles is getting enough quality data. Many healthcare systems struggle with this, especially if it’s a rarer disease and they only have a few dozen patients. To develop reliable models, you need hundreds, thousands, and sometimes tens of thousands of dataset points/patients. Collecting that data in a high-quality manner, and of course with proper approvals and safeguards in place, is one of the most difficult tasks when creating digital biomarkers. Additionally, there’s still no established FDA regulation regarding AI technologies, so although the conversation has started, it will be a while before widespread adoption occurs.
Taking it a step further, once the necessary regulatory approvals have been granted, how do we get digital biomarkers into the healthcare system? All technical aspects must be integrated effectively into existing IT ecosystems. We also have to consider who will be paying for this new technology. Payers are not used to these platforms yet so it will be crucial that developers of digital biomarkers demonstrate the important cost savings these tools could deliver for healthcare systems.
Joshua Reicher: Things in healthcare tend to move slowly, but I am sure we will get there in the end. Just look at the field of genetics and genomics. They were really interesting research concepts in the early 1990s. Today, they are both universally covered for a wide range of different use cases in clinical practice across the country, by every payer. Over a period of 10 to 15 years, we went from having neither genetics nor genomic testing available to having all of these processes – from regulatory to reimbursement to use in the clinical setting – relatively streamlined.
Any new technology is going to go through growing pains, but we’re optimistic about the adoption of digital biomarkers because of the range of benefits they offer for everyone in the healthcare system.
HT: As engineer-physicians, what are your thoughts on the significant growth of deep technologies, such as AI and machine learning, and how they will continue to merge with biology to change the healthcare sector?
Michael Muelly: The complexity of data on a population level is getting increasingly difficult to manage. These technologies are playing a crucial role in helping us to find meaningful correlations among vast amounts of data. The insights we are gaining are enabling us to develop a better understanding of individual patient differences. This level of knowledge is helping physicians to devise ever more personalized treatment plans
Unfortunately, there continues to be an overpromise of AI and machine learning. There is a lot that these technologies still cannot achieve. For example, they will not be able to replace doctors for the vast majority of tasks. For the industry, it is best to take a more grounded and realistic perspective on what these technologies can do and the value they add.
Implementing digital biomarkers: How executives can adopt new solutions
HT: Have you seen a positive shift where healthcare providers are more open to digital solutions? In that regard, what are the challenges that healthcare providers face when wanting to implement digital biomarkers?
Michael Muelly: There’s certainly more understanding around digital solutions these days. The enthusiasm around AI has been increasing over the last 10 years, and we have also seen some fairly sophisticated technological advances. These digital technologies are also increasingly accepted as stakeholders begin to realize how helpful these tools can be.
If healthcare systems are to adopt digital biomarkers, stakeholders need to ask several questions.
First is “how can we ensure that these devices actually work well?” Health networks must measure the value that these digital biomarkers generate, and guarantee that their safety is up to the highest standard.
The next step is to ask “is there enough incentive for the healthcare system to cover the costs of these technologies, or will they be reimbursed directly?” Even after gauging the value of these devices, there are still doubts as to their costs and payment coverage.
Lastly, “how can we integrate these technologies into our current processes?” Digital developers must first get a good grasp of the present operations within a specific health system, and then figure out how best to introduce and include these new digital biomarker technologies in order to provide their anticipated value.
HT: Let’s further discuss the topic of healthcare organization implementation. If a company like IMVARIA is approaching a healthcare organization with its digital biomarker solution, what is the best approach for getting the technology integrated into its provided services?
Michael Muelly: That’s a big challenge – you have to figure out the stakeholders who can make that decision. We think about it in two different ways. One is the physician-focused approach, where, if there is payer coverage similar to a send-out lab test, the physician is ultimately the one who makes the decision to order the diagnostic solution. In that case, there is one person who needs to be convinced of the value of the technology. When rolling out the technology on a more healthcare systems level for an entire organization, it becomes a healthcare leadership decision with a different value proposition.
The complexity of rolling a technology out at the healthcare system level is significantly higher compared to convincing one individual clinician. While you can touch a lot more lives at the healthcare systems level, getting into these environments can take years. It is best to pursue a dual approach, where we want to make this technology available to individual clinicians who could have patients benefit from it, but also pursue that avenue of wide adoption at the healthcare systems level.
Joshua Reicher: In the liquid biopsy space, there are two models to consider when thinking about digital biomarkers. The first model is to show that the biomarker is linked to diagnosis accuracy or outcome, determine payment for it through health economic studies, and then clinicians can access it without any extra integration. They just need to submit the data and get the results back. The second model involves working with biopharmaceutical and life sciences companies, clinical trials, and sites interested in particular diseases to implement technologies for earlier diagnoses or for trial enrollment. This precedes the need for regulatory authorization since it is done under clinical research review boards, and helps get patients into studies more quickly while also providing potentially quicker diagnoses in that context.
Insights for healthcare executives for digital biomarker opportunities
HT: To wrap up our conversation, can you provide three use cases for digital biomarker implementation and how they can make a difference for healthcare organizations?
Joshua Reicher & Michael Muelly:
Automated systems are proving to be useful in identifying rare and challenging diseases that may have otherwise gone unrecognized. This automation allows us to prioritize these cases, and we see this as a great opportunity for the future.
Clinical trial enrollment and assessment is another potential use for digital biomarkers; this will help speed things up and make the evaluations more objective and consistent. This is a major issue now because sponsors are running trials at various clinical sites with multiple clinicians assessing the patients. Anything that can be done to ensure consistent enrollment criteria and evaluation of responses across sites would be beneficial.
Digital biomarkers can also be used as diagnostics tools to reduce misdiagnosis, underdiagnosis, and incorrect phenotyping. These technologies provide clinicians with more reliable performance metrics when dealing with rare cases, which are often overlooked or misidentified. Digital biomarkers can help physicians accurately differentiate rare cases from the more common ones.
- U.S. Food and Drug Administration. (2022). Article avaliable from https://www.fda.gov/patients/rare-diseases-fda [Accessed February 2023]
- Nord Rare Insights. (2020). Report available from https://rarediseases.org/wp-content/uploads/2022/10/NRD-2088-Barriers-30-Yr-Survey-Report_FNL-2.pdf [Accessed February 2023]
- Society for Improve Diganosis in Medicine. (2018). Report available from https://www.improvediagnosis.org/roadmap-for-research-to-improve-diagnosis-public-health-imperative/ [Accessed February 2023]
- Hoyer et al. (2019). Respiratory Research 20. Paper available from https://respiratory-research.biomedcentral.com/articles/10.1186/s12931-019-1076-0 [Accessed February 2023]
- Richeldi et al. (2012). Thorax 67, 407-411. Paper available from https://pubmed.ncbi.nlm.nih.gov/22426899/ Supplementary figures available from https://thorax.bmj.com/content/thoraxjnl/suppl/2012/03/16/thoraxjnl-2011-201184.DC1/thoraxjnl-2011-201184-s1.pdf [Accessed February 2023]
- Chiu , Kao , Simoff, et al. (2021). CEOR 13, 191-200. Paper available from https://pubmed.ncbi.nlm.nih.gov/33762834/ [Accessed February 2023]
- Imvaria. (2023). Data on file
- Heartflow. Webpage available from https://www.heartflow.com/ [Accessed February 2023]
- Lim. (2016). Nat Rev Cardiology 13. Paper available from https://www.nature.com/articles/nrcardio.2016.123 [Accessed February 2023]